forked from PulseFocusPlatform/PulseFocusPlatform
Compare commits
5 Commits
setchanged
...
master
Author | SHA1 | Date |
---|---|---|
![]() |
21a36f151a | |
![]() |
e6485ffebb | |
![]() |
726c07fb9c | |
![]() |
b3ce719d97 | |
|
ea96f9ae05 |
117
README.md
117
README.md
|
@ -5,7 +5,8 @@ Pulse Focus Platform脉冲聚焦是面向水底物体图像识别的实时检测
|
||||||
脉冲聚焦软件设计了图片和视频两种数据输入下的多物体识别功能。针对图片数据,调用模型进行单张图片预测,随后在前端可视化输出多物体识别结果;针对视频流动态图像数据,首先对视频流数据进行分帧采样,获取采样图片,再针对采样图片进行多物体识别,将采样识别结果进行视频合成,然后在前端可视化输出视频流数据识别结果。为了视频流数据处理的高效性,设计了采样-识别-展示的多线程处理方式,可加快视频流数据处理。
|
脉冲聚焦软件设计了图片和视频两种数据输入下的多物体识别功能。针对图片数据,调用模型进行单张图片预测,随后在前端可视化输出多物体识别结果;针对视频流动态图像数据,首先对视频流数据进行分帧采样,获取采样图片,再针对采样图片进行多物体识别,将采样识别结果进行视频合成,然后在前端可视化输出视频流数据识别结果。为了视频流数据处理的高效性,设计了采样-识别-展示的多线程处理方式,可加快视频流数据处理。
|
||||||
|
|
||||||
软件界面简单,易学易用,包含参数的输入选择,程序的运行,算法结果的展示等,源代码公开,算法可修改。
|
软件界面简单,易学易用,包含参数的输入选择,程序的运行,算法结果的展示等,源代码公开,算法可修改。
|
||||||
开发人员:K. Wang、H.P. Yu、J. Li、H.T. Li、Z.Q. Wang、Z.Y. Zhao、L.F. Zhang、G. Chen
|
|
||||||
|
开发人员:K. Wang、H.P. Yu、J. Li、Z.Y. Zhao、L.F. Zhang、G. Chen、H.T. Li、Z.Q. Wang、Y.G. Han
|
||||||
|
|
||||||
## 1. 开发环境配置
|
## 1. 开发环境配置
|
||||||
运行以下命令:
|
运行以下命令:
|
||||||
|
@ -21,11 +22,119 @@ conda env create -f create_env.yaml
|
||||||
python main.py
|
python main.py
|
||||||
```
|
```
|
||||||
|
|
||||||
## 3. 一些说明
|
## 3. 软硬件运行平台
|
||||||
1. 使用GPU版本
|
|
||||||
|
(1)配置要求
|
||||||
|
|
||||||
|
<table>
|
||||||
|
<tr>
|
||||||
|
<th>组件</th>
|
||||||
|
<th>配置</th>
|
||||||
|
<th>备注</th>
|
||||||
|
</tr>
|
||||||
|
<tr>
|
||||||
|
<td>系统 </td>
|
||||||
|
<td>Windows 10 家庭中文版 20H2 64位</td>
|
||||||
|
<td>扩展支持Linux和Mac系统</td>
|
||||||
|
</tr>
|
||||||
|
<tr>
|
||||||
|
<td>处理器</td>
|
||||||
|
<td>处理器类型:
|
||||||
|
酷睿i3兼容处理器或速度更快的处理器
|
||||||
|
处理器速度:
|
||||||
|
最低:1.0GHz
|
||||||
|
建议:2.0GHz或更快
|
||||||
|
</td>
|
||||||
|
<td>不支持ARM、IA64等芯片处理器</td>
|
||||||
|
</tr>
|
||||||
|
<tr>
|
||||||
|
<td>内存</td>
|
||||||
|
<td>RAM 16.0 GB (15.7 GB 可用)</td>
|
||||||
|
<td></td>
|
||||||
|
</tr>
|
||||||
|
<tr>
|
||||||
|
<td>显卡</td>
|
||||||
|
<td>最小:核心显卡
|
||||||
|
推荐:GTX1060或同类型显卡
|
||||||
|
</td>
|
||||||
|
<td></td>
|
||||||
|
</tr>
|
||||||
|
<tr>
|
||||||
|
<td>硬盘</td>
|
||||||
|
<td>500G</td>
|
||||||
|
<td></td>
|
||||||
|
</tr>
|
||||||
|
<td>显示器</td>
|
||||||
|
<td>3840×2160像素,高分屏</td>
|
||||||
|
<td></td>
|
||||||
|
</tr>
|
||||||
|
<tr>
|
||||||
|
</tr>
|
||||||
|
<td>软件</td>
|
||||||
|
<td>Anaconda3 2020及以上</td>
|
||||||
|
<td>Python3.7及以上,需手动安装包</td>
|
||||||
|
</tr>
|
||||||
|
</table>
|
||||||
|
(2)手动部署及运行
|
||||||
|
|
||||||
|
推荐的安装步骤如下:
|
||||||
|
|
||||||
|
安装Anaconda3-2020.02-Windows-x86_64或以上版本;
|
||||||
|
手动安装pygame、pymunk、pyyaml、numpy、easydict和pyqt,安装方式推荐参考如下:
|
||||||
|
```
|
||||||
|
pip install -i https://pypi.tuna.tsinghua.edu.cn/simple pygame==2.0.1
|
||||||
|
```
|
||||||
|
将软件模块文件夹拷贝到电脑中(以D盘为例,路径为D:\island-multi_ships)
|
||||||
|
|
||||||
|
## 4. 软件详细介绍
|
||||||
|
软件总体开发系统架构图如下所示。
|
||||||
|
|
||||||
|

|
||||||
|
(1)界面设计
|
||||||
|
平台界面设计如上图所示,界面各组件功能设计如下:
|
||||||
|
|
||||||
|

|
||||||
|
|
||||||
|
* 静态图像导入:用于选择需要进行预测的单张图像,可支持jpg,png,jpeg等格式图像,选择图像后,会在下方界面进行展示。
|
||||||
|
* 动态图像导入:用于选择需要进行预测的单个视频,可支持pm4等格式视频,选择视频后,会在下方界面进行展示。
|
||||||
|
* 信息导出:用于在预测完成后,将预测后的照片,视频导出到具体文件夹下。
|
||||||
|
* 特征选择:由于挑选相关特征。
|
||||||
|
* 预处理方法:由于选择相关预处理方法。
|
||||||
|
* 识别算法:用于选择预测时的所需算法,目前支持YOLO与RCNN两种模型算法。
|
||||||
|
* GPU加速:选择是否使用GPU进行预测加速,对视频预测加速效果明显。
|
||||||
|
* 识别:当相关配置完成后,点击识别选项,会进行预测处理,并将预测后的视频或图像在下方显示。
|
||||||
|
* 训练:目前考虑到GPU等资源限制,未完整开放。
|
||||||
|
* 信息显示:在界面右下角显示类别flv,gx,mbw,object的识别目标个数。
|
||||||
|
|
||||||
|
2)主要功能设计
|
||||||
|
|
||||||
|
设计了图片和视频两种数据输入的多目标识别功能。针对图片数据,调用模型进行单张图片预测,随后在前端可视化输出多目标识别结果;针对视频流动态图像数据,首先对视频流数据进行分帧采样,获取采样图片,再针对采样图片进行多目标识别,将采样识别结果进行视频合成,然后在前段可视化输出视频流数据识别结果。为求视频流数据处理的高效性,设计了采样-识别-展示的多线性处理方式,可加快视频流数据处理。
|
||||||
|
* 侧扫声呐图像多目标识别功能
|
||||||
|
* 侧扫声呐视频多目标识别功能
|
||||||
|
## 5. 软件使用结果
|
||||||
|
Faster-RCNN模型在四种目标物图片上的识别验证结果如下所示:
|
||||||
|
|
||||||
|

|
||||||
|
|
||||||
|
|
||||||
|
YOLOV3模型在四种目标物图片上的识别验证结果如下所示:
|
||||||
|
|
||||||
|
|
||||||
|

|
||||||
|
|
||||||
|
PP-YOLO-BOT模型在四种目标物图片上的识别验证结果如下所示:
|
||||||
|
|
||||||
|

|
||||||
|
|
||||||
|
调用PP-YOLO-BOT模型对视频数据进行识别验证,结果如下截图所示:
|
||||||
|
|
||||||
|
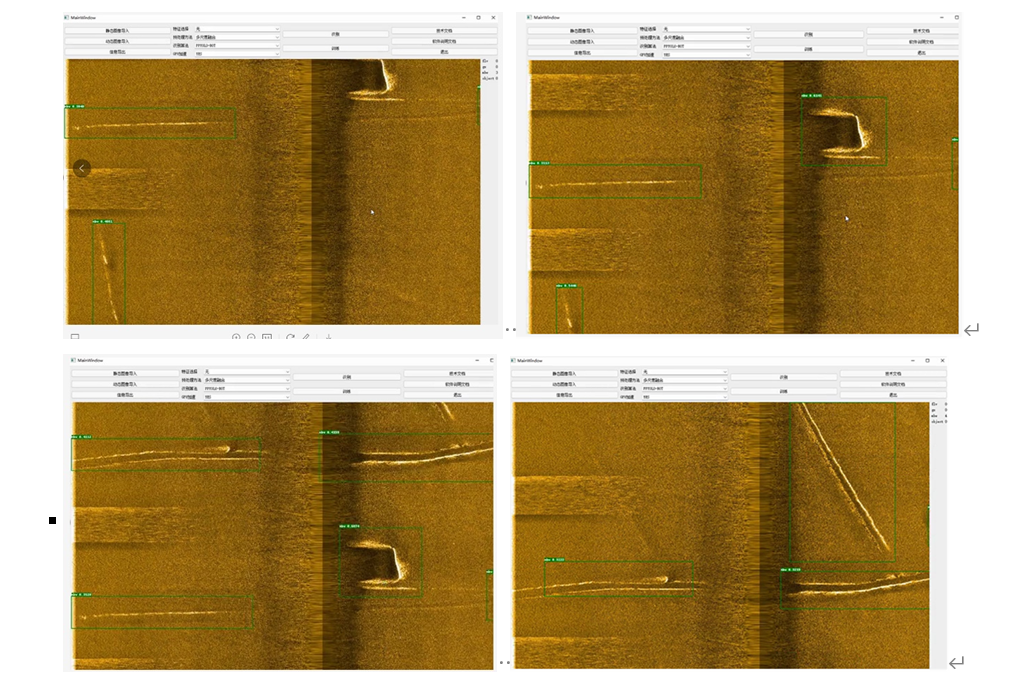
|
||||||
|
|
||||||
|
## 6. 其他说明
|
||||||
|
* 使用GPU版本
|
||||||
|
|
||||||
参考百度飞桨paddle官方网站安装
|
参考百度飞桨paddle官方网站安装
|
||||||
|
|
||||||
[安装链接](https://www.paddlepaddle.org.cn/install/quick?docurl=/documentation/docs/zh/install/pip/windows-pip.html)
|
[安装链接](https://www.paddlepaddle.org.cn/install/quick?docurl=/documentation/docs/zh/install/pip/windows-pip.html)
|
||||||
|
|
||||||
2. 模型文件全部更新在inference_model中,pic为测试图片
|
* 模型文件全部更新在inference_model中,pic为测试图片
|
||||||
|
|
1
main.py
1
main.py
|
@ -68,6 +68,7 @@ class mywindow(QtWidgets.QMainWindow, Ui_MainWindow):
|
||||||
print('cd {}'.format(self.path1))
|
print('cd {}'.format(self.path1))
|
||||||
print(
|
print(
|
||||||
'python deploy/python/infer.py --model_dir={} --video_file={} --use_gpu=True'.format(self.model_path, self.Video_fname))
|
'python deploy/python/infer.py --model_dir={} --video_file={} --use_gpu=True'.format(self.model_path, self.Video_fname))
|
||||||
|
# 调用GPU
|
||||||
os.system('cd {}'.format(self.path1))
|
os.system('cd {}'.format(self.path1))
|
||||||
os.system(
|
os.system(
|
||||||
'python deploy/python/infer.py --model_dir={} --image_dir={} --output_dir=./video_output/{} --threshold=0.3 --use_gpu=True'.format(
|
'python deploy/python/infer.py --model_dir={} --image_dir={} --output_dir=./video_output/{} --threshold=0.3 --use_gpu=True'.format(
|
||||||
|
|
Binary file not shown.
After Width: | Height: | Size: 26 KiB |
Binary file not shown.
After Width: | Height: | Size: 19 KiB |
Binary file not shown.
After Width: | Height: | Size: 360 KiB |
Binary file not shown.
After Width: | Height: | Size: 350 KiB |
Binary file not shown.
After Width: | Height: | Size: 372 KiB |
Binary file not shown.
After Width: | Height: | Size: 942 KiB |
Loading…
Reference in New Issue