Compare commits
No commits in common. "master" and "master" have entirely different histories.
|
@ -1,5 +1,5 @@
|
|||
[bumpversion]
|
||||
current_version = 0.1.0
|
||||
current_version = 0.0.1
|
||||
commit = True
|
||||
tag = True
|
||||
tag_name = v{new_version}
|
||||
|
|
41
README.md
41
README.md
|
@ -7,7 +7,10 @@
|
|||
[](https://hub.docker.com/r/idrl/idrlnet)
|
||||
[](https://www.codefactor.io/repository/github/idrl-lab/idrlnet/overview/master)
|
||||
|
||||
**IDRLnet** is a machine learning library on top of [PyTorch](https://pytorch.org/). Use IDRLnet if you need a machine learning library that solves both forward and inverse differential equations via physics-informed neural networks (PINN). IDRLnet is a flexible framework inspired by [Nvidia Simnet](https://developer.nvidia.com/simnet>).
|
||||
|
||||
**IDRLnet** is a machine learning library on top of [PyTorch](https://pytorch.org/). Use IDRLnet if you need a machine
|
||||
learning library that solves both forward and inverse differential equations via physics-informed neural
|
||||
networks (PINN). IDRLnet is a flexible framework inspired by [Nvidia Simnet](https://developer.nvidia.com/simnet>).
|
||||
|
||||
## Docs
|
||||
|
||||
|
@ -63,27 +66,21 @@ pip install -e .
|
|||
|
||||
IDRLnet supports
|
||||
|
||||
- complex domain geometries without mesh generation. Provided geometries include interval, triangle, rectangle, polygon, circle, sphere... Other geometries can be constructed using three boolean operations: union, difference, and intersection;
|
||||
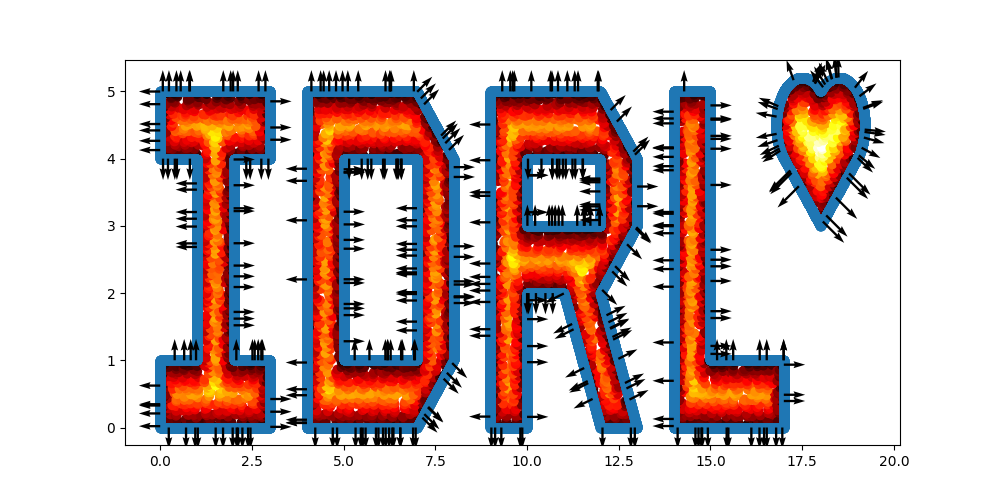
|
||||
|
||||
- sampling in the interior of the defined geometry or on the boundary with given conditions.
|
||||
- complex domain geometries without mesh generation. Provided geometries include interval, triangle, rectangle, polygon,
|
||||
circle, sphere... Other geometries can be constructed using three boolean operations: union, difference, and
|
||||
intersection;
|
||||
|
||||
- enables the user code to be structured. Data sources, operations, constraints are all represented by ``Node``. The graph will be automatically constructed via label symbols of each node. Getting rid of the explicit construction via explicit expressions, users model problems more naturally.
|
||||
- sampling in the interior of the defined geometry or on the boundary with given conditions.
|
||||
|
||||
- builds computational graph automatically;
|
||||
- enables the user code to be structured. Data sources, operations, constraints are all represented by ``Node``. The graph
|
||||
will be automatically constructed via label symbols of each node. Getting rid of the explicit construction via
|
||||
explicit expressions, users model problems more naturally.
|
||||
|
||||

|
||||
|
||||
- user-defined callbacks;
|
||||
|
||||

|
||||
|
||||
- solving variational minimization problem;
|
||||
<img src="https://raw.githubusercontent.com/weipeng0098/picture/master/20210617082331.gif" alt="miniface" style="zoom:33%;" />
|
||||
|
||||
- solving integral differential equation;
|
||||
|
||||
- adaptive resampling;
|
||||
- solving integral differential equation;
|
||||
|
||||
- adaptive resampling;
|
||||
|
||||
- recover unknown parameters of PDEs from noisy measurement data.
|
||||
|
||||
|
@ -102,10 +99,12 @@ First off, thanks for taking the time to contribute!
|
|||
|
||||
- **Reporting bugs.** To report a bug, simply open an issue in the GitHub "Issues" section.
|
||||
|
||||
- **Suggesting enhancements.** To submit an enhancement suggestion for IDRLnet, including completely new features and minor improvements to existing functionality, let us know by opening an issue.
|
||||
|
||||
- **Pull requests.** If you made improvements to IDRLnet, fixed a bug, or had a new example, feel free to send us a pull-request.
|
||||
|
||||
- **Suggesting enhancements.** To submit an enhancement suggestion for IDRLnet, including completely new features and
|
||||
minor improvements to existing functionality, let us know by opening an issue.
|
||||
|
||||
- **Pull requests.** If you made improvements to IDRLnet, fixed a bug, or had a new example, feel free to send us a
|
||||
pull-request.
|
||||
|
||||
- **Asking questions.** To get help on how to use IDRLnet or its functionalities, you can as well open an issue.
|
||||
|
||||
- **Answering questions.** If you know the answer to any question in the "Issues", you are welcomed to answer.
|
||||
|
|
|
@ -22,7 +22,7 @@ copyright = "2021, IDRL"
|
|||
author = "IDRL"
|
||||
|
||||
# The full version, including alpha/beta/rc tags
|
||||
release = "0.1.0"
|
||||
release = "0.0.1"
|
||||
|
||||
# -- General configuration ---------------------------------------------------
|
||||
|
||||
|
|
|
@ -3,7 +3,7 @@ Consider the 1d wave equation:
|
|||
|
||||
$$
|
||||
\begin{equation}
|
||||
\frac{\partial^2u}{\partial t^2}=c^2\frac{\partial^2u}{\partial x^2},
|
||||
\frac{\partial^2u}{\partial t^2}=c\frac{\partial^2u}{\partial x^2},
|
||||
\end{equation}
|
||||
$$
|
||||
where $c>0$ is unknown and is to be estimated. A group of data pairs $\{x_i, t_i, u_i\}_{i=1,2,\cdot,N}$ is observed.
|
||||
|
@ -11,7 +11,7 @@ Then the problem is formulated as:
|
|||
|
||||
$$
|
||||
\min_{u,c} \sum_{i=1,2,\cdots,N} \|u(x_i, t_i)-u_i\|^2\\
|
||||
s.t. \frac{\partial^2u}{\partial t^2}=c^2\frac{\partial^2u}{\partial x^2}
|
||||
s.t. \frac{\partial^2u}{\partial t^2}=c\frac{\partial^2u}{\partial x^2}
|
||||
$$
|
||||
|
||||
In the context of PINN, $u$ is parameterized to $u_\theta$.
|
||||
|
@ -20,7 +20,7 @@ The problem above is transformed to the discrete form:
|
|||
$$
|
||||
\min_{\theta,c}
|
||||
w_1\sum_{i=1,2,\cdots,N} \|u_\theta(x_i, t_i)-u_i\|^2
|
||||
+w_2\sum_{i=1,2,\cdots,M}\left|\frac{\partial^2u_\theta(x_i,t_i)}{\partial t^2}-c^2\frac{\partial^2u_\theta(x_i,t_i)}{\partial x^2}\right|^2.
|
||||
+w_2\sum_{i=1,2,\cdots,M}\left|\frac{\partial^2u_\theta(x_i,t_i)}{\partial t^2}-c\frac{\partial^2u_\theta(x_i,t_i)}{\partial x^2}\right|^2.
|
||||
$$
|
||||
|
||||
## Importing External Data
|
||||
|
|
|
@ -1,25 +0,0 @@
|
|||
import idrlnet.shortcut as sc
|
||||
import math
|
||||
import sympy as sp
|
||||
import numpy as np
|
||||
import matplotlib.pyplot as plt
|
||||
|
||||
order = 10
|
||||
s = 0
|
||||
h = 1
|
||||
cv = 0.6
|
||||
x, t = sp.symbols('x t')
|
||||
|
||||
for k in range(1, order + 1):
|
||||
s += (-1) ** (k - 1) / (2 * k - 1) * sp.cos((2 * k - 1) * math.pi * x / 2 / h) * sp.exp(
|
||||
-(2 * k - 1) ** 2 * math.pi ** 2 / 4 * cv * t / h / h)
|
||||
p_ratio = s * 4 / math.pi
|
||||
p_ratio_fn = sc.lambdify_np(p_ratio, ['t', 'x'])
|
||||
|
||||
if __name__ == '__main__':
|
||||
t_num = np.linspace(0.01, 1, 100, endpoint=True)
|
||||
z_num = np.linspace(0.01, 1, 100, endpoint=True)
|
||||
tt_num, zz_num = np.meshgrid(t_num, z_num)
|
||||
p_ratio_num = p_ratio_fn(tt_num, zz_num)
|
||||
plt.scatter(tt_num, zz_num, c=p_ratio_num, cmap='jet', vmin=0, vmax=1)
|
||||
plt.show()
|
|
@ -1,124 +0,0 @@
|
|||
import idrlnet.shortcut as sc
|
||||
import sympy as sp
|
||||
import numpy as np
|
||||
import matplotlib.pyplot as plt
|
||||
from mpl_toolkits.axes_grid1 import make_axes_locatable
|
||||
from Aana import p_ratio_fn
|
||||
|
||||
cv = 0.6
|
||||
|
||||
x, y = sp.symbols('x y')
|
||||
p = sp.Function('p')(x, y)
|
||||
geo = sc.Rectangle((0, 0), (1, 1))
|
||||
|
||||
|
||||
@sc.datanode
|
||||
class Init(sc.SampleDomain):
|
||||
def __init__(self):
|
||||
self.points = geo.sample_boundary(100, sieve=(sp.Eq(y, 0.)))
|
||||
self.constraints = {'p': 1., 'lambda_p': 1 - np.power(self.points['x'], 5)}
|
||||
|
||||
def sampling(self, *args, **kwargs):
|
||||
return self.points, self.constraints
|
||||
|
||||
|
||||
@sc.datanode
|
||||
class Interior(sc.SampleDomain):
|
||||
def __init__(self):
|
||||
self.points = geo.sample_interior(10000)
|
||||
self.constraints = {'consolidation': np.zeros_like(self.points['x'])}
|
||||
|
||||
def sampling(self, *args, **kwargs):
|
||||
return self.points, self.constraints
|
||||
|
||||
|
||||
@sc.datanode
|
||||
class UpperBounds(sc.SampleDomain):
|
||||
def __init__(self):
|
||||
self.points = geo.sample_boundary(100, sieve=(sp.Eq(x, 1.)))
|
||||
self.constraints = {'p': 0.,
|
||||
'lambda_p': np.power(self.points['y'], 0.2)}
|
||||
|
||||
def sampling(self, *args, **kwargs):
|
||||
return self.points, self.constraints
|
||||
|
||||
|
||||
@sc.datanode
|
||||
class LowerBounds(sc.SampleDomain):
|
||||
def __init__(self):
|
||||
self.points = geo.sample_boundary(100, sieve=(sp.Eq(x, 0.)))
|
||||
self.constraints = {'normal_gradient_p': 0., }
|
||||
|
||||
def sampling(self, *args, **kwargs):
|
||||
return self.points, self.constraints
|
||||
|
||||
|
||||
pde = sc.ExpressionNode(name='consolidation', expression=p.diff(y) - cv * p.diff(x, 2))
|
||||
grad = sc.NormalGradient(T='p', dim=2, time=False)
|
||||
net = sc.get_net_node(inputs=('x', 'y'), outputs=('p',), arch=sc.Arch.mlp, name='net')
|
||||
|
||||
s = sc.Solver(sample_domains=(Init(), Interior(), UpperBounds(), LowerBounds()),
|
||||
netnodes=[net],
|
||||
pdes=[pde, grad],
|
||||
max_iter=2000)
|
||||
s.solve()
|
||||
|
||||
|
||||
@sc.datanode
|
||||
class MeshInterior(sc.SampleDomain):
|
||||
def sampling(self, *args, **kwargs):
|
||||
t_num = np.linspace(0.01, 1, 400, endpoint=True)
|
||||
z_num = np.linspace(0.01, 1, 100, endpoint=True)
|
||||
tt_num, zz_num = np.meshgrid(t_num, z_num)
|
||||
points = {'x': zz_num.reshape(-1, 1), 'y': tt_num.reshape(-1, 1)}
|
||||
return points, {}
|
||||
|
||||
|
||||
s.sample_domains = (MeshInterior(),)
|
||||
points = s.infer_step({'MeshInterior': ['x', 'y', 'p']})
|
||||
x_num = points['MeshInterior']['x'].detach().cpu().numpy().ravel()
|
||||
y_num = points['MeshInterior']['y'].detach().cpu().numpy().ravel()
|
||||
p_num = points['MeshInterior']['p'].detach().cpu().numpy().ravel()
|
||||
|
||||
_, ax = plt.subplots(3, 1, figsize=(10, 10))
|
||||
|
||||
im = ax[0].scatter(y_num, x_num, c=p_num, vmin=0, vmax=1, cmap='jet')
|
||||
ax[0].set_xlim([0, 1.01])
|
||||
ax[0].set_ylim([-0.05, 1.05])
|
||||
ax[0].set_ylabel('z(m)')
|
||||
divider = make_axes_locatable(ax[0])
|
||||
cax = divider.append_axes('right', size='2%', pad=0.1)
|
||||
plt.colorbar(im, cax=cax)
|
||||
ax[0].set_title('Model Prediction: $p_{pred}=p/p_0(z,t)$')
|
||||
|
||||
ax[1].set_xlim([0, 1.01])
|
||||
ax[1].set_ylim([-0.05, 1.05])
|
||||
ax[1].set_ylabel('z(m)')
|
||||
ax[1].set_title('Ground Truth: $p_{true}=p/p_0(z,t)$')
|
||||
t_num = np.linspace(0.01, 1, 400, endpoint=True)
|
||||
z_num = np.linspace(0.01, 1, 100, endpoint=True)
|
||||
tt_num, zz_num = np.meshgrid(t_num, z_num)
|
||||
|
||||
p_ratio_num = p_ratio_fn(tt_num, zz_num)
|
||||
|
||||
im = ax[1].scatter(tt_num, zz_num, c=p_ratio_num, cmap='jet', vmin=0, vmax=1.)
|
||||
divider = make_axes_locatable(ax[1])
|
||||
cax = divider.append_axes('right', size='2%', pad=0.1)
|
||||
plt.colorbar(im, cax=cax)
|
||||
|
||||
im = ax[2].scatter(tt_num, zz_num, c=p_num.reshape(100, 400) - p_ratio_num, cmap='bwr', vmin=-1,
|
||||
vmax=1)
|
||||
ax[2].set_xlim([0, 1.01])
|
||||
ax[2].set_ylim([-0.05, 1.05])
|
||||
ax[2].set_xlabel('t(yr)')
|
||||
ax[2].set_ylabel('z(m)')
|
||||
ax[2].set_title('Error: $p_{pred}-p_{true}$')
|
||||
|
||||
divider = make_axes_locatable(ax[2])
|
||||
cax = divider.append_axes('right', size='2%', pad=0.1)
|
||||
cbar = plt.colorbar(im, cax=cax)
|
||||
|
||||
plt.tight_layout(pad=0.4, w_pad=0.5, h_pad=1.0)
|
||||
plt.savefig('test.png')
|
||||
plt.show()
|
||||
plt.close()
|
|
@ -1,2 +0,0 @@
|
|||
`Aana.py` generate the ground truth via truncated analytical expression.
|
||||
`Boneside.py` solves the consolidation equation.
|
|
@ -1,234 +0,0 @@
|
|||
import idrlnet.shortcut as sc
|
||||
import torch
|
||||
import matplotlib.pyplot as plt
|
||||
import numpy as np
|
||||
import sympy as sp
|
||||
import abc
|
||||
from matplotlib import tri
|
||||
|
||||
sc.use_gpu(device=0)
|
||||
|
||||
INTERVAL = 10000
|
||||
DENSITY = 10000
|
||||
|
||||
r1, r2, r3, r4, r5, r6 = sp.symbols('r1 r2 r3 r4 r5 r6')
|
||||
|
||||
plate = sc.Tube2D((-1, -0.5), (1, 0.5))
|
||||
hole_1 = sc.Circle(center=(-0.6, 0), radius=r1)
|
||||
hole_2 = sc.Circle(center=(0., 0.), radius=r2)
|
||||
hole_3 = sc.Circle(center=(0.5, -0.5), radius=r3)
|
||||
hole_4 = sc.Circle(center=(0.5, 0.5), radius=r4)
|
||||
hole_5 = sc.Circle(center=(-0.5, -0.5), radius=r5)
|
||||
hole_6 = sc.Circle(center=(-0.5, 0.5), radius=r6)
|
||||
geo = plate - hole_1 - hole_2 - hole_3 - hole_4 - hole_5 - hole_6
|
||||
|
||||
in_line = sc.Line((-1, -0.5), (-1, 0.5), normal=1)
|
||||
out_line = sc.Line((1, -0.5), (1, 0.5), normal=1)
|
||||
param_ranges = {r1: (0.05, 0.2),
|
||||
r2: (0.05, 0.2),
|
||||
r3: (0.05, 0.2),
|
||||
r4: (0.05, 0.2),
|
||||
r5: (0.05, 0.2),
|
||||
r6: (0.05, 0.2), }
|
||||
|
||||
|
||||
class ReSampleDomain(sc.SampleDomain, metaclass=abc.ABCMeta):
|
||||
"""
|
||||
Resampling collocated points every INTERVAL iterations.
|
||||
"""
|
||||
count = 0
|
||||
points = sc.Variables()
|
||||
constraints = sc.Variables()
|
||||
|
||||
def sampling(self, *args, **kwargs):
|
||||
if self.count % INTERVAL == 0:
|
||||
self.do_re_sample()
|
||||
sc.logger.info("Resampling...")
|
||||
self.count += 1
|
||||
return self.points, self.constraints
|
||||
|
||||
@abc.abstractmethod
|
||||
def do_re_sample(self):
|
||||
pass
|
||||
|
||||
|
||||
@sc.datanode
|
||||
class InPlane(ReSampleDomain):
|
||||
def do_re_sample(self):
|
||||
self.points = sc.Variables(
|
||||
in_line.sample_boundary(param_ranges=param_ranges, density=DENSITY,
|
||||
low_discrepancy=True)).to_torch_tensor_()
|
||||
self.constraints = {'T': torch.ones_like(self.points['x'])}
|
||||
|
||||
|
||||
@sc.datanode
|
||||
class OutPlane(ReSampleDomain):
|
||||
def do_re_sample(self):
|
||||
self.points = sc.Variables(
|
||||
out_line.sample_boundary(param_ranges=param_ranges, density=DENSITY,
|
||||
low_discrepancy=True)).to_torch_tensor_()
|
||||
self.constraints = {'T': torch.zeros_like(self.points['x'])}
|
||||
|
||||
|
||||
@sc.datanode(sigma=10.)
|
||||
class Boundary(ReSampleDomain):
|
||||
def do_re_sample(self):
|
||||
self.points = sc.Variables(
|
||||
geo.sample_boundary(param_ranges=param_ranges, density=DENSITY, low_discrepancy=True)).to_torch_tensor_()
|
||||
self.constraints = {'normal_gradient_T': torch.zeros_like(self.points['x'])}
|
||||
|
||||
|
||||
@sc.datanode
|
||||
class Interior(ReSampleDomain):
|
||||
def do_re_sample(self):
|
||||
self.points = sc.Variables(
|
||||
geo.sample_interior(param_ranges=param_ranges, density=DENSITY, low_discrepancy=True)).to_torch_tensor_()
|
||||
self.constraints = {'diffusion_T': torch.zeros_like(self.points['x'])}
|
||||
|
||||
|
||||
net = sc.get_net_node(inputs=('x', 'y', 'r1', 'r2', 'r3', 'r4', 'r5', 'r6'), outputs=('T',), name='net',
|
||||
arch=sc.Arch.mlp_xl)
|
||||
pde = sc.DiffusionNode(T='T', D=1., Q=0, dim=2, time=False)
|
||||
grad = sc.NormalGradient('T', dim=2, time=False)
|
||||
|
||||
s = sc.Solver(sample_domains=(InPlane(), OutPlane(), Boundary(), Interior()),
|
||||
netnodes=[net],
|
||||
pdes=[pde, grad],
|
||||
max_iter=100000,
|
||||
schedule_config=dict(scheduler='ExponentialLR', gamma=0.99998))
|
||||
|
||||
s.solve()
|
||||
|
||||
|
||||
# Define inference domains.
|
||||
|
||||
@sc.datanode
|
||||
class Inference(sc.SampleDomain):
|
||||
def sampling(self, *args, **kwargs):
|
||||
self.points = sc.Variables(
|
||||
geo.sample_interior(param_ranges=param_ranges, density=20000, low_discrepancy=True)).to_torch_tensor_()
|
||||
self.constraints = {'T__x': torch.zeros_like(self.points['x']),
|
||||
'T__y': torch.zeros_like(self.points['x']), }
|
||||
return self.points, self.constraints
|
||||
|
||||
|
||||
@sc.datanode
|
||||
class BoundaryInference(sc.SampleDomain):
|
||||
def sampling(self, *args, **kwargs):
|
||||
self.points = sc.Variables(
|
||||
geo.sample_boundary(param_ranges=param_ranges, density=1000, low_discrepancy=True)).to_torch_tensor_()
|
||||
self.constraints = {'T__x': torch.zeros_like(self.points['x']),
|
||||
'T__y': torch.zeros_like(self.points['x']), }
|
||||
return self.points, self.constraints
|
||||
|
||||
|
||||
@sc.datanode
|
||||
class InPlane(sc.SampleDomain):
|
||||
def sampling(self, *args, **kwargs):
|
||||
self.points = sc.Variables(
|
||||
in_line.sample_boundary(param_ranges=param_ranges, density=1000,
|
||||
low_discrepancy=True)).to_torch_tensor_()
|
||||
self.constraints = {'T__x': torch.zeros_like(self.points['x']),
|
||||
'T__y': torch.zeros_like(self.points['x']), }
|
||||
return self.points, self.constraints
|
||||
|
||||
|
||||
@sc.datanode
|
||||
class OutPlane(sc.SampleDomain):
|
||||
def sampling(self, *args, **kwargs):
|
||||
self.points = sc.Variables(
|
||||
out_line.sample_boundary(param_ranges=param_ranges, density=1000,
|
||||
low_discrepancy=True)).to_torch_tensor_()
|
||||
self.constraints = {'T__x': torch.zeros_like(self.points['x']),
|
||||
'T__y': torch.zeros_like(self.points['x']), }
|
||||
return self.points, self.constraints
|
||||
|
||||
|
||||
s.sample_domains = (InPlane(), OutPlane(), Inference(), BoundaryInference())
|
||||
|
||||
count = [0]
|
||||
|
||||
|
||||
def parameter_design(*args):
|
||||
"""
|
||||
Do inference and plot the result.
|
||||
"""
|
||||
|
||||
param_ranges[r1] = args[0]
|
||||
param_ranges[r2] = args[1]
|
||||
param_ranges[r3] = args[2]
|
||||
param_ranges[r4] = args[3]
|
||||
param_ranges[r5] = args[4]
|
||||
param_ranges[r6] = args[5]
|
||||
|
||||
points = s.infer_step({'Inference': ['x', 'y', 'T__x', 'T__y', ],
|
||||
'BoundaryInference': ['x', 'y', 'T__x', 'T__y', ],
|
||||
'InPlane': ['x', 'y', 'T__x', 'T__y', ],
|
||||
'OutPlane': ['x', 'y', 'T__x', 'T__y', ]})
|
||||
|
||||
plt.figure(figsize=(8, 4))
|
||||
fig = plt.gcf()
|
||||
fig.set_tight_layout(True)
|
||||
########
|
||||
num_x = points['BoundaryInference']['x'].detach().cpu().numpy().ravel()
|
||||
num_y = points['BoundaryInference']['y'].detach().cpu().numpy().ravel()
|
||||
|
||||
num_T__x = points['BoundaryInference']['T__x'].detach().cpu().numpy().ravel()
|
||||
num_T__y = points['BoundaryInference']['T__y'].detach().cpu().numpy().ravel()
|
||||
|
||||
num_flux = np.sqrt(num_T__x ** 2 + num_T__y ** 2)
|
||||
plt.scatter(x=num_x, y=num_y, c=num_flux, s=3, vmin=0, vmax=0.8, cmap='bwr')
|
||||
|
||||
########
|
||||
num_x = points['InPlane']['x'].detach().cpu().numpy().ravel()
|
||||
num_y = points['InPlane']['y'].detach().cpu().numpy().ravel()
|
||||
|
||||
num_T__x = points['InPlane']['T__x'].detach().cpu().numpy().ravel()
|
||||
num_T__y = points['InPlane']['T__y'].detach().cpu().numpy().ravel()
|
||||
|
||||
num_flux = np.sqrt(num_T__x ** 2 + num_T__y ** 2)
|
||||
plt.scatter(x=num_x, y=num_y, c=num_flux, s=3, vmin=0, vmax=0.8, cmap='bwr')
|
||||
|
||||
########
|
||||
num_x = points['OutPlane']['x'].detach().cpu().numpy().ravel()
|
||||
num_y = points['OutPlane']['y'].detach().cpu().numpy().ravel()
|
||||
|
||||
num_T__x = points['OutPlane']['T__x'].detach().cpu().numpy().ravel()
|
||||
num_T__y = points['OutPlane']['T__y'].detach().cpu().numpy().ravel()
|
||||
|
||||
num_flux = np.sqrt(num_T__x ** 2 + num_T__y ** 2)
|
||||
plt.scatter(x=num_x, y=num_y, c=num_flux, s=3, vmin=0, vmax=0.8, cmap='bwr')
|
||||
|
||||
########
|
||||
num_x = points['Inference']['x'].detach().cpu().numpy().ravel()
|
||||
num_y = points['Inference']['y'].detach().cpu().numpy().ravel()
|
||||
|
||||
num_T__x = points['Inference']['T__x'].detach().cpu().numpy().ravel()
|
||||
num_T__y = points['Inference']['T__y'].detach().cpu().numpy().ravel()
|
||||
|
||||
num_flux = np.sqrt(num_T__x ** 2 + num_T__y ** 2)
|
||||
points['Inference']['T_flux'] = num_flux
|
||||
|
||||
triang = tri.Triangulation(num_x, num_y)
|
||||
|
||||
def apply_mask(triang, alpha=0.4):
|
||||
triangles = triang.triangles
|
||||
xtri = num_x[triangles] - np.roll(num_x[triangles], 1, axis=1)
|
||||
ytri = num_y[triangles] - np.roll(num_y[triangles], 1, axis=1)
|
||||
maxi = np.max(np.sqrt(xtri ** 2 + ytri ** 2), axis=1)
|
||||
triang.set_mask(maxi > alpha)
|
||||
|
||||
apply_mask(triang, alpha=0.04)
|
||||
plt.tricontourf(triang, num_flux, 100, vmin=0, vmax=0.8, cmap='bwr')
|
||||
|
||||
ax = plt.gca()
|
||||
ax.set_facecolor('k')
|
||||
ax.set_xticks([])
|
||||
ax.set_yticks([])
|
||||
ax.set_xlim([-1, 1.])
|
||||
ax.set_ylim([-0.5, 0.5])
|
||||
plt.savefig("holes.png")
|
||||
plt.close()
|
||||
|
||||
|
||||
parameter_design(0.14, 0.1, 0.2, 0.09, 0.05, 0.17)
|
|
@ -1,115 +0,0 @@
|
|||
from idrlnet import shortcut as sc
|
||||
import sympy as sp
|
||||
import torch
|
||||
import numpy as np
|
||||
from idrlnet.pde_op.equations import NavierStokesNode
|
||||
from matplotlib import tri
|
||||
import matplotlib.pyplot as plt
|
||||
|
||||
x, y = sp.symbols('x y')
|
||||
rec = sc.Rectangle((-0.05, -0.05), (0.05, 0.05))
|
||||
|
||||
|
||||
@sc.datanode(name='flow_domain')
|
||||
class InteriorDomain(sc.SampleDomain):
|
||||
def __init__(self):
|
||||
points = rec.sample_interior(400000, bounds={x: (-0.05, 0.05), y: (-0.05, 0.05)})
|
||||
|
||||
constraints = sc.Variables({'continuity': torch.zeros(len(points['x']), 1),
|
||||
'momentum_x': torch.zeros(len(points['x']), 1),
|
||||
'momentum_y': torch.zeros(len(points['x']), 1)})
|
||||
|
||||
points['area'] = np.ones_like(points['area']) * 2.5e-6
|
||||
|
||||
constraints['lambda_continuity'] = points['sdf']
|
||||
constraints['lambda_momentum_x'] = points['sdf']
|
||||
constraints['lambda_momentum_y'] = points['sdf']
|
||||
self.points = sc.Variables(points).to_torch_tensor_()
|
||||
self.constraints = sc.Variables(constraints).to_torch_tensor_()
|
||||
|
||||
def sampling(self, *args, **kwargs):
|
||||
return self.points, self.constraints
|
||||
|
||||
|
||||
@sc.datanode(name='left_right_down')
|
||||
class LeftRightDownBoundaryDomain(sc.SampleDomain):
|
||||
def __init__(self):
|
||||
points = rec.sample_boundary(3333, sieve=(y < 0.05))
|
||||
constraints = sc.Variables({'u': torch.zeros(len(points['x']), 1), 'v': torch.zeros(len(points['x']), 1)})
|
||||
points['area'] = np.ones_like(points['area']) * 1e-4
|
||||
|
||||
self.points = sc.Variables(points).to_torch_tensor_()
|
||||
self.constraints = sc.Variables(constraints).to_torch_tensor_()
|
||||
|
||||
def sampling(self, *args, **kwargs):
|
||||
return self.points, self.constraints
|
||||
|
||||
|
||||
@sc.datanode(name='up')
|
||||
class UpBoundaryDomain(sc.SampleDomain):
|
||||
def __init__(self):
|
||||
points = rec.sample_boundary(10000, sieve=sp.Eq(y, 0.05))
|
||||
points['area'] = np.ones_like(points['area']) * 1e-4
|
||||
constraints = sc.Variables({'u': torch.ones(len(points['x']), 1), 'v': torch.zeros(len(points['x']), 1)})
|
||||
constraints['lambda_u'] = 1 - 20 * abs(points['x'].copy())
|
||||
self.points = sc.Variables(points).to_torch_tensor_()
|
||||
self.constraints = sc.Variables(constraints).to_torch_tensor_()
|
||||
|
||||
def sampling(self, *args, **kwargs):
|
||||
return self.points, self.constraints
|
||||
|
||||
|
||||
torch.autograd.set_detect_anomaly(True)
|
||||
net = sc.MLP([2, 100, 100, 100, 100, 3], activation=sc.Activation.tanh, initialization=sc.Initializer.Xavier_uniform,
|
||||
weight_norm=False)
|
||||
|
||||
net_u = sc.NetNode(inputs=('x', 'y',), outputs=('u', 'v', 'p'), net=net, name='net_u')
|
||||
pde = NavierStokesNode(nu=0.01, rho=1.0, dim=2, time=False)
|
||||
|
||||
s = sc.Solver(sample_domains=(InteriorDomain(), LeftRightDownBoundaryDomain(), UpBoundaryDomain()),
|
||||
netnodes=[net_u],
|
||||
pdes=[pde],
|
||||
max_iter=4000,
|
||||
network_dir='./result/tanh_Xavier_uniform',
|
||||
)
|
||||
s.solve()
|
||||
|
||||
|
||||
def interoir_domain_infer():
|
||||
points = rec.sample_interior(1000000, bounds={x: (-0.05, 0.05), y: (-0.05, 0.05)})
|
||||
constraints = sc.Variables({'continuity': torch.zeros(len(points['x']), 1),
|
||||
'momentum_x': torch.zeros(len(points['x']), 1),
|
||||
'momentum_y': torch.zeros(len(points['x']), 1)})
|
||||
return points, constraints
|
||||
|
||||
|
||||
data_infer = sc.get_data_node(interoir_domain_infer, name='flow_domain')
|
||||
s.sample_domains = [data_infer]
|
||||
|
||||
pred = s.infer_step({'flow_domain': ['x', 'y', 'v', 'u', 'p']})
|
||||
num_x = pred['flow_domain']['x'].detach().cpu().numpy().ravel()
|
||||
num_y = pred['flow_domain']['y'].detach().cpu().numpy().ravel()
|
||||
num_u = pred['flow_domain']['u'].detach().cpu().numpy().ravel()
|
||||
num_v = pred['flow_domain']['v'].detach().cpu().numpy().ravel()
|
||||
num_p = pred['flow_domain']['p'].detach().cpu().numpy().ravel()
|
||||
triang_total = tri.Triangulation(num_x, num_y)
|
||||
|
||||
triang_total = tri.Triangulation(num_x, num_y)
|
||||
u_pre = num_u.flatten()
|
||||
|
||||
fig = plt.figure(figsize=(15, 5))
|
||||
ax1 = fig.add_subplot(131)
|
||||
tcf = ax1.tricontourf(triang_total, num_u, 100, cmap="jet")
|
||||
tc_bar = plt.colorbar(tcf)
|
||||
ax1.set_title('u')
|
||||
|
||||
ax2 = fig.add_subplot(132)
|
||||
tcf = ax2.tricontourf(triang_total, num_v, 100, cmap="jet")
|
||||
tc_bar = plt.colorbar(tcf)
|
||||
ax2.set_title('v')
|
||||
|
||||
ax3 = fig.add_subplot(133)
|
||||
tcf = ax3.tricontourf(triang_total, num_p, 100, cmap="jet")
|
||||
tc_bar = plt.colorbar(tcf)
|
||||
ax3.set_title('p')
|
||||
plt.show()
|
|
@ -1,43 +1,16 @@
|
|||
import torch
|
||||
from .header import logger
|
||||
|
||||
GPU_AVAILABLE = False
|
||||
GPU_ENABLED = False
|
||||
# todo more careful check
|
||||
GPU_ENABLED = True
|
||||
if torch.cuda.is_available():
|
||||
try:
|
||||
_ = torch.Tensor([0.0, 0.0]).cuda()
|
||||
logger.info("GPU available")
|
||||
GPU_AVAILABLE = True
|
||||
torch.set_default_tensor_type("torch.cuda.FloatTensor")
|
||||
print("gpu available")
|
||||
GPU_ENABLED = True
|
||||
except:
|
||||
logger.info("GPU not available")
|
||||
GPU_AVAILABLE = False
|
||||
print("gpu not available")
|
||||
GPU_ENABLED = False
|
||||
else:
|
||||
logger.info("GPU not available")
|
||||
GPU_AVAILABLE = False
|
||||
|
||||
|
||||
def use_gpu(device=0):
|
||||
"""Use GPU with device `device`.
|
||||
|
||||
Args:
|
||||
device (torch.device or int): selected device.
|
||||
"""
|
||||
if GPU_AVAILABLE:
|
||||
try:
|
||||
torch.cuda.set_device(device)
|
||||
torch.set_default_tensor_type("torch.cuda.FloatTensor")
|
||||
logger.info(f"Using GPU device {device}")
|
||||
global GPU_ENABLED
|
||||
GPU_ENABLED = True
|
||||
except:
|
||||
logger.warning("Invalid device ordinal")
|
||||
|
||||
|
||||
def use_cpu():
|
||||
"""
|
||||
Use CPU.
|
||||
"""
|
||||
if GPU_ENABLED:
|
||||
torch.set_default_tensor_type("torch.FloatTensor")
|
||||
logger.info(f"Using CPU")
|
||||
print("gpu not available")
|
||||
GPU_ENABLED = False
|
||||
|
|
|
@ -223,7 +223,7 @@ def get_data_nodes(funs: List[Callable], *args, **kwargs) -> Tuple[DataNode]:
|
|||
|
||||
|
||||
class SampleDomain(metaclass=abc.ABCMeta):
|
||||
"""The Template for Callable sampling functions."""
|
||||
"""Template for Callable sampling function."""
|
||||
|
||||
@abc.abstractmethod
|
||||
def sampling(self, *args, **kwargs):
|
||||
|
|
|
@ -4,7 +4,7 @@ import math
|
|||
from math import pi
|
||||
from typing import Union, List, Tuple
|
||||
import numpy as np
|
||||
from sympy import symbols, Abs, sqrt, Max, Min, cos, sin, log, sign, Heaviside, asin
|
||||
from sympy import symbols, Abs, sqrt, Max, Min, cos, sin, log, sign, Heaviside
|
||||
from sympy.vector import CoordSys3D
|
||||
from .geo import Edge, Geometry1D, Geometry2D, Geometry3D
|
||||
|
||||
|
@ -662,21 +662,11 @@ class Box(Geometry3D):
|
|||
|
||||
class Sphere(Geometry3D):
|
||||
def __init__(self, center, radius):
|
||||
x, y, z, v_1, v_2 = symbols("x y z v_1 v_2")
|
||||
ranges = {v_1: (0, 1), v_2: (0, 1)}
|
||||
|
||||
theta = 2 * pi * v_1
|
||||
r = sqrt(v_2)
|
||||
|
||||
phi = 2 * asin(r) # latitude
|
||||
r_1 = cos(theta) * sin(phi)
|
||||
r_2 = sin(theta) * sin(phi)
|
||||
r_3 = cos(phi)
|
||||
# x, y, z, v_1, v_2, u_1, u_2 = symbols("x y z v_1 v_2 u_1 u_2")
|
||||
# ranges = {v_1: (0, 1), v_2: (0, 1), u_1: (0, 1), u_2: (0, 1)}
|
||||
# r_1 = sqrt(-log(v_1)) * cos(2 * pi * u_1)
|
||||
# r_2 = sqrt(-log(v_1)) * sin(2 * pi * u_1)
|
||||
# r_3 = sqrt(-log(v_2)) * cos(2 * pi * u_2)
|
||||
x, y, z, v_1, v_2, u_1, u_2 = symbols("x y z v_1 v_2 u_1 u_2")
|
||||
ranges = {v_1: (0, 1), v_2: (0, 1), u_1: (0, 1), u_2: (0, 1)}
|
||||
r_1 = sqrt(-log(v_1)) * cos(2 * pi * u_1)
|
||||
r_2 = sqrt(-log(v_1)) * sin(2 * pi * u_1)
|
||||
r_3 = sqrt(-log(v_2)) * cos(2 * pi * u_2)
|
||||
|
||||
norm = sqrt(r_1 ** 2 + r_2 ** 2 + r_3 ** 2)
|
||||
edge_1 = Edge(
|
||||
|
|
|
@ -10,4 +10,4 @@ from idrlnet.callbacks import GradientReceiver
|
|||
from idrlnet.receivers import Receiver, Signal
|
||||
from idrlnet.variable import Variables, export_var
|
||||
from idrlnet.header import logger
|
||||
from idrlnet import GPU_AVAILABLE, GPU_ENABLED, use_gpu
|
||||
from idrlnet import GPU_ENABLED
|
||||
|
|
|
@ -18,5 +18,4 @@ pyevtk==1.1.1
|
|||
flask==1.1.2
|
||||
requests==2.25.0
|
||||
torch>=1.7.1
|
||||
networkx==2.5.1
|
||||
protobuf~=3.20
|
||||
networkx==2.5.1
|
2
setup.py
2
setup.py
|
@ -21,7 +21,7 @@ def load_requirements(path_dir=here, comment_char="#"):
|
|||
|
||||
setuptools.setup(
|
||||
name="idrlnet", # Replace with your own username
|
||||
version="0.1.0",
|
||||
version="0.0.1",
|
||||
author="Intelligent Design & Robust Learning lab",
|
||||
author_email="weipeng@deepinfar.cn",
|
||||
description="IDRLnet",
|
||||
|
|
Loading…
Reference in New Issue